The main focus for KMC is to develop a platform to aggregate and integrate data from various sources to illuminate knowledge that together with machine learning algorithms and expert curation could inspire scientists to seek and explore new associations within the human proteome.
KMC grant at UNM is led by Dr. Tudor Oprea and includes collaborations with Dr. Lars Juhl Jensen’s lab at NNFCPR and at EMBL-EBI with Dr. Andrew Leach as their Group Lead. Work from UNM includes the development of TCRD, the main database supporting Pharos, and appropriate visualization tools, such as TINx.
As a partner with the KMC, NCATS is developing and implementing PHAROS as the User Interface Portal to access all the integrated data, metadata and annotation collected into TCRD and via tools from ISMMS.
EMBL-EBI has developed tools for automated extraction and expert curation of medicinal chemistry data. The group led by A. Leach has extracted pertinent target-chemical pairs from the patent literature and late-stage drug development, as well as the clinical candidate literature.
Work by Dr. Lars Juhl Jensen has resulted in improved text-mining technology that is applied to scientific literature for scoring how well studied each target protein may be and to support target prioritization. This text-mining platform also helps provide tissue and disease associations for the targets.
The KMC at ISMMS is led by Dr. Avi Ma’ayan. Through systematic data integration and application of machine learning methods, the Ma'ayan Lab is filling knowledge gaps about the understudied IDG targets of interest. The Harmonizome which some of it is incorporated into Pharos, provides access to all integrated data and methods.
DRGC – Data and Resource Generation Centers (NIH RFA-RM-16-026)
The objective of the DRGCs is to further illuminate understudied targets in the three main druggable protein families: G-protein coupled receptors, ion channels, and protein kinases. Their experimental workflows incorporate multiple robust assays on cellular basis of target action and extends to animal models. Data and Resources generated are disseminated via RDOC.
GPCR
Collaborative work by Dr. Bryan Roth and Dr. Brian Shoichet focuses on illuminating the druggable GPCR-ome by a two-pronged approach of empirical screening of drugs followed by computational screening against modeled structures of the GPCR to produce optimized lead compounds. Their work has led to discovery of molecule “ogerin” binding to previously orphaned GPR68.
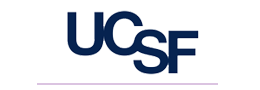
Ion Channels
Dr. Michael T. McManus together with Dr. Lily Jan leads a group researchers from UCSF and UCD focused on illuminating Ion Channels by utilizing CRISPR technology to map expression profiles, assess channel activities, develop antibodies, and generate new mouse lines.
Kinases
Dr. Gary L. Johnson has established a network of collaborators; Dr. Shawn Gomez (UNC), Dr. Ben Major (UNC), Dr. Tim Willson (SGC), Dr. Reid Townsend (WU), and Dr. Peter Sorger (HMS), to tackle the illumination of function of the understudied druggable kinome. Their work includes technologies of Multiplex Inhibitor Beads (MIB) / Mass Spectrometry (MS) to identify kinase activation status in response to perturbants, and applies results to model cell lines and patient derived xenographs.
CEITs will develop and deploy tools to enhance the community's ability to process, analyze, and visualize IDG data, prioritize new data resources and methods to be incorporated into Pharos and develop methods to prioritize understudied IDG families.
Collaborative work by Dr. Kannan and Dr. Kochut will further the development of Protein Kinase Ontology (ProKinO) for organizing information about kinase structure, function and evolution in order to apply to the visualization of the understudied kinases in these organizational context. .
Dr. Wu, Dr. Stein, and Dr. D’Eustachio will extend their work on REACTOME knowledgebase, a biological pathway database, for illuminating interactions of IDG proteins with other proteins and biological entities with the aim to develop a special Reactome IDG pathway portal allowing for evaluation of these understudied proteins via their localization, potential interactions, and developing testable predictions.

The CEIT-JAX group will develop a knowledge graphs for elucidating further understanding of the IDG target proteins via collections of information from Monarch Initiative and Drug Central and then deploy machine learning algorithms with the intent to develop testable hypothesis for evaluating the functions of understudied kinases.
This CEIT, led by Dr. Kannan and Dr. Lu, will focus on ion channels and develop cutting edge tools and resources that cater to the unique needs of the ion channel community.
The collaboration of Dr. Pandey and Dr. Schlessinger will develop a novel computational framework to predict kinase-substrate interactions by combining biologically relevant multi-modal data sources with cutting-edge machine learning methodologies.
This CEIT aims to integrate the recently available cancer proteogenomics data from the Clinical Proteomic Tumor Analysis Consortium (CPTAC) into the characterization of the understudied proteins in these families.